Ontology in AI: Meaning, Example, Types, Use-application. In Artificial Intelligence, ontology relates to a shared vocabulary for researchers. It includes definitions of the basic concept and the relation between them which are machine-interpretable.
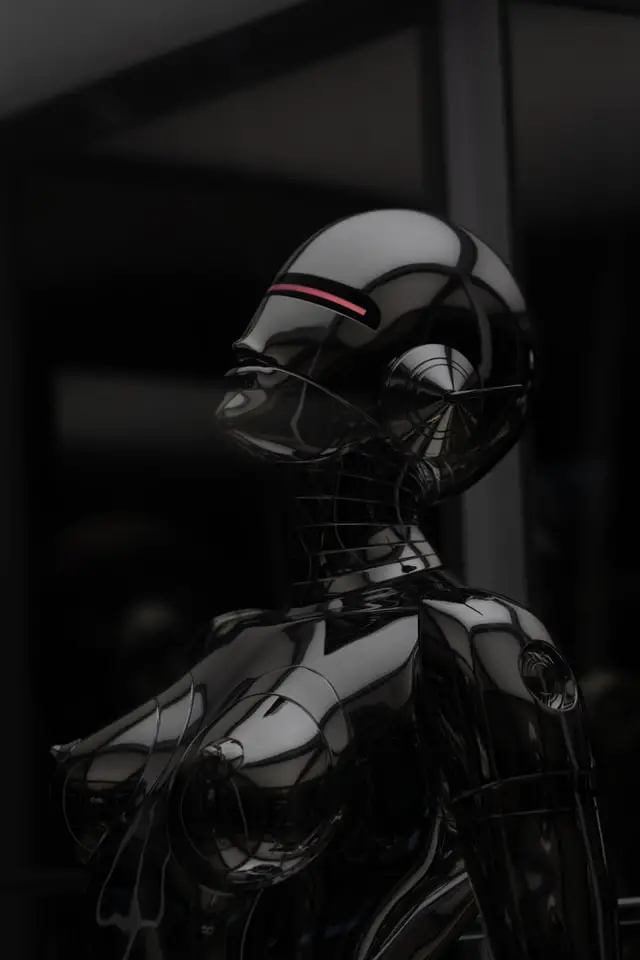
Ontology in AI allows the commands of the system to use the contents and relationships between them to make the speculation that imitates human behavior.
If talking about ontology alone then it is a branch of philosophy that deals with the study of existence and being. Moreover, practical business refers to construction that binds different sources of information and consists of interconnecting data from multiple domains. It can be used as a means of resolving organizational differences to enhance integration between databases.
Meaning
Let’s have a look at the meaning of ontology in Artificial Intelligence. Rapid advancement has been seen in Artificial Intelligence and its branches such as deep learning, and machine learning in the previous years.
The work of Artificial Intelligence is that it extracts relevant information and generates insights from data to find suitable solutions which have been observed throughout the discovery of Artificial Intelligence.
But the running of these programs is not easy as it requires algorithms, data, and code, and its translation into something meaningful requires data science.
With the help of data sciences, organizations can communicate with customers, stakeholders, track, and analyze the trend. Presence of Ontology in this case with relevant terms and connections from a specific domain the process of identifying the concept, improving the concept, and unifying of data for critical information becomes streamlined.
A common misconception is that machine learning gets better with more data however many researchers have denied this claim. With stepping forward the businesses realize that too much can be overwhelming to analyze, destroying the complexity of the value and higher investments in terms of money and time both.
According to the studies, 85% of projects of Artificial Intelligence fail because of this reason. It is because of a lack of understanding of how a large amount of data can be utilized. If we focus them in this direction, the ontologies mark the remarkable differential.
Ontological modeling can help an AI system to broaden its scope. It can include any type of data and whether the data is in a structured, unstructured, or unstructured format. It smooths the data integration. It can give a vast amount of data as input. The benefit of ontology is that it can diverse organizations in different industries setting out unique goals.
According to Enterprises, AI should be able to allow faster digital transformation means the ability to rapidly scale is essential. Companies can enable this by developing a repeatable framework instead of using a chain of data in one location that could grow through existing associative relationships.
For organizations to power, their AI projects Ontology can help provide a reusable, and adaptive structure.
Example
Ontology-based Artificial Intelligence can result in extremely targeted results and it does not require training sets to become functional as well. Regarding AI, different ontologies exist that can be considered as examples.
For instance, a natural processing system can make use of ontologies to decide that the word cat and dog are semantically similar. Two of the prominent ontologies are present in Ontolingua and Cyc. An abstract layer of Wordnet (lexical database) can be considered as a simple ontology.
Ontology-based AI emulates human performance and allows the system to make inferences based on content and relationships.
Types
Knowledge models are of different types that run along a continuum beginning from the simplest level in which a controlled vocabulary is introduced for the encouragement of the use of the same for a particular meaning. There are different types of ontologies that are used in Artificial Intelligence. Some of them are:
The Semantic Web
The semantic web is a way through which machine-interpretable knowledge is allowed to be distributed on the Web. These pages are also meant to be read by humans instead of just providing HTML pages. These websites also provide information that can be used by computers.
RDF
RDF allows the sentences to be reified in their languages which means it can be presenting arbitrary logical formulas which are generally undecidable. To be undecidable is not necessarily a bad thing, it is just that computation time that the system may take cannot be fixed. For instance, simple logic programs with function symbols, and all the programming languages are virtually undecidable.
XML
XML or Extensive Markup language is used for providing a machine-readable syntax design. Moreover, humans can read it too. It is a text-based language in which items are placed hierarchically. The syntax for XML is quite complicated however at the simple level, the tag is either in the form <tag…/>.
URI
A URI or Uniform Resource Identifier is used for the unique identification of a resource. A resource can be anything carrying a unique identity. A URI is a string referring to a resource that may include a person, a web page, or a corporation however commonly URIs use the syntax of a web address.
Use-Application: Ontology in AI
In Artificial Intelligence Ontology has widespread uses as it helps to improve the quality of data for training datasets. It provides more coherent and easy navigation when users wish to move from one concept to another in ontology structure.
On the other hand, interestingly ontology can be used for the creation of a knowledge graph for the set of individual facts. A piece of knowledge can be described as a set of entities where nodes and edges between the nodes explain the type and relationship between them.
A recent uptake has been seen in expressing ontologies with the use of ontology in languages such as Web Ontology Language (OWL). A domain-specific ontology is a combination with AI-driven tools for data analytics which can serve relevant data and uncover new data trends and patterns. It means that ontology can fit every organization’s goal which can either be logical, semantic, mathematical based approaches.
I hope you like the article, share it as it will be a gesture of appreciation for us.
Read also: Ontology in Information Science ; IT Ethics; Ontology of education
External resource: earley
This post is also available in:
English
Français (French)
Deutsch (German)
Español (Spanish)
Dansk (Danish)
Nederlands (Dutch)
Svenska (Swedish)
Italiano (Italian)
Português (Portuguese (Portugal))